
Jack Schroder
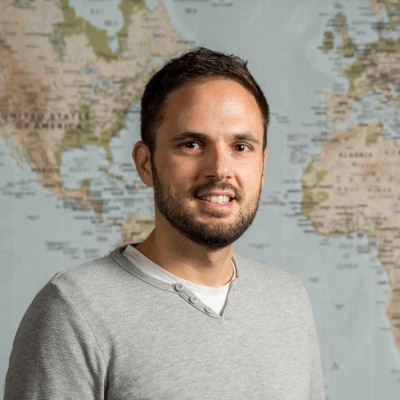
Miguel Alvarez
•
July 8, 2020
Last updated
•
min read
Originally posted by CARTO.
As the impact of the COVID-19 pandemic continues to be felt across all industries, particularly retail, there are both ongoing record declines as well as some encouraging signs of recovery depending on location.
For example in May total retail sales fell a huge 52.1% in Singapore whereas the eurozone’s largest economy Germany reported a record rise of 13.9% following the easing of restrictions. As we have reported on previously these differences can be reflected by the unequal nature of the virus’ spread. Regardless it has become clear that in order for retailers to survive and optimize response to the recovery they must invest in technology and explore new sources of consumer data.
With the recent integration of Spatial.ai’s geosocial data in CARTO’s Data Observatory, our customers are now able to uniquely explore a wealth of relationships and patterns between behavioral data and that from other categories such as financial, human mobility and demographics; unveiling new insights about a location. In this case study, we have explored how geosocial data interacts with credit card transaction data, in order to understand the relationships between social media behaviors and restaurant sales. The results live up to the hype; illuminating relevant market differences that can help retailers optimize their footprint depending on the territory.
With social media now being the speaker through which people express their feelings, opinions and interests into the world; it has also become a great platform to source valuable information about a location and how people behave when being around it. Spatial.ai has turned all that vast amount of information generated through posts, tweets, and stories into a comprehensive set of 70+ social segments.
At the same time, aggregated credit card transaction patterns are key for understanding consumer behaviors and how those evolve over time and space. Mastercard Geographic Insights provides highly granular metrics to measure the evolution of the consumer expenditure in a retail area, allowing for validation, evaluation and benchmarking of the sales-based dynamics of a location.
For this analysis, we were interested not only in the relationships between credit card transactions and geosocial data, but also in how these relationships change across different markets. To investigate these questions, we selected data for the metropolitan areas of Los Angeles and Chicago to explore their commonalities and differences.
To begin we looked at credit card spend data alone in order to discover the top locations for restaurant sales.
As shown in the above maps, top performers in Chicago concentrate mainly in the downtown area. However, in LA they are spread throughout the city. It is also interesting to see how in Chicago underperformers are far away from top performers, while in LA they all mix in nearby areas.
For our analysis, we are most interested in the areas that capture the most restaurant sales. What are these areas like? Thankfully, geosocial data can help us to quantitatively answer this question. The following charts show aggregated geosocial scores for the areas where the restaurants with higher credit card spend are located in both cities.
Based on the charts, you can see that the areas with the top restaurant spend share the same social segments: LGBTQ Culture, Wealth Signaling, Men’s Style, and Wine Lovers. These segments are interesting; together, they tell a story of trendiness, urbanicity, and wealth.
There are, of course, some differences in the top segments. As we might expect to see in LA, Film Lovers is showing up as a top segment. These differences hint that perhaps different segments are positive indicators of restaurant success in each market. To figure this out, the best step is to look at correlations between geosocial segments and restaurant sales data. The following chart shows the 35 Geosocial Segments with the strongest correlations (for any of the cities) and with the highest differences between the two cities. They are sorted by the correlation coefficient for Chicago.
There is a lot of information wrapped up in this chart. A lot more than we can get into in this post. Let’s focus though on two main learnings that we want to point out.
If one looks at the segments Film Lovers, LGBTQ Culture, and Yoga Advocates; for each of these variables, their correlations with restaurant sales are much higher in LA than in Chicago. While most segments tend to either be positive or negative for both markets, a couple actually differ. For example, Party Life has a moderately strong positive relationship with sales in LA but a negative correlation in Chicago.
The segments that performed relatively well in Chicago were focused on proximity to beautiful landmarks and landscapes. Both Sites to See and Natural Beauty had positive relationships with sales in Chicago but not LA (Outdoor Adventures also follows the pattern, but not as strongly).
These differences have serious implications for location decisions in the two cities. Based on these results, it doesn’t necessarily make sense to use the same segments to choose restaurant locations in the two cities.
Perhaps the most interesting pattern we saw in these results was how segments related to behaviors that are sometimes seen as controversial, or even counter-cultural, seemed to perform better in LA than Chicago. Namely, the segments Body Art, Hip Hop Culture, Hipster, Party Life, and Activism all showed (to varying degrees) higher correlation with restaurant sales in LA than in Chicago.
Correlation does not imply causation. But, when viewing results like these ones, it is useful and interesting to hypothesize about why the data shows what it shows. In this case, we hypothesize that perhaps the progressive culture of LA is more broadly accepting of the listed behaviors, thereby leading to these behaviors being less likely to negatively impact restaurant sales.
The relationships between geosocial data and credit card transactions reveal that people’s mindsets, interests, and attitudes correlate with the sales potential at a location. And using only demographic data as part of an analysis misses the larger picture of the uniqueness of each community. It’s clear from this analysis that while restaurant sales in LA and Chicago share a number of characteristics that drive success, there are also differences. And when these differences are not or cannot be taken into account, output will likely be suboptimal.
By jointly analyzing geosocial data and financial data, it’s possible to glean quantified insights that only a local would know. In Chicago, it was as easy as correlating restaurant sales data with geosocial variables to see that proximity to Natural Beauty and important landmarks are great indicators of restaurant success. This may seem obvious, but it is very difficult to quantify in other ways. Further, note that the same relationship is not nearly as strong in LA.
Want to start incorporating data from Spatial.ai and Mastercard, and other high-quality datasets into your models and spatial analyses?